October 2024
Learn and Search: From Data to Discovery
Learning
&
Discovery
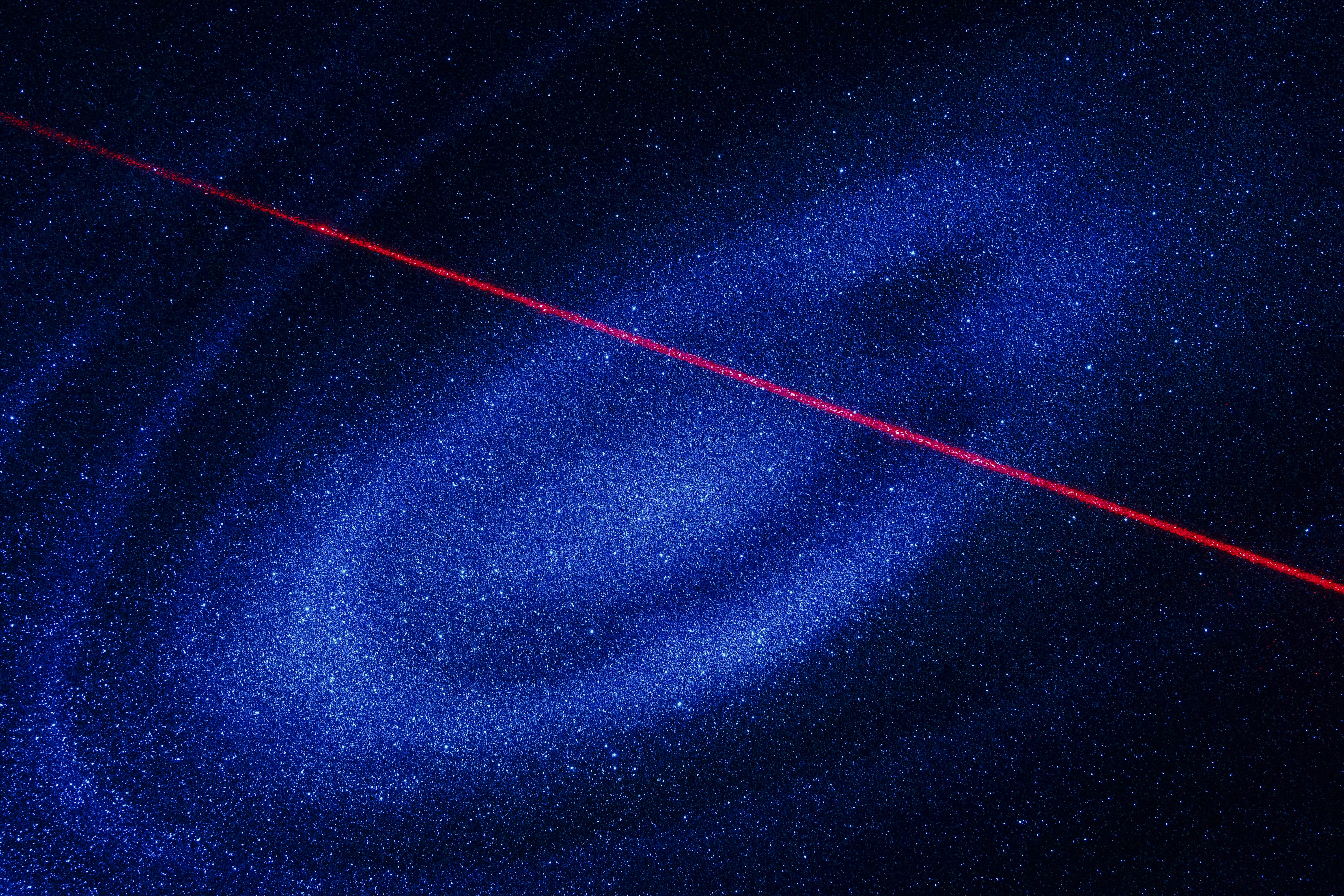
Listen to the Podcast Version
Introduction
How do we go from learning from our data to discovering new sciences and technological breakthroughs that help us understand the universe?
Richard Sutton, a leading researcher in AI, famously articulated "The Bitter Lesson": that learning and search are the two approaches that scale without limits. It implies that, to make significant progress, we need systems capable of learning from vast data sources and applying search techniques to discover new insights.
Learn from Data and Search for Solutions
ChatGPT showed us that learning from diverse datasets is possible with enough data, computational power, and model capacity. In a sense, we have now learned from almost all human-acquired knowledge—everything transmitted, shared, and written on the internet. It has taken billions of years for natural selection and human evolution to accumulate this knowledge, but today, we can speed up the learning process with intelligent machines.
Machines are not constrained biologically. They benefit from faster computation, parallel processing, and consistent hardware capabilities. Where human brains have evolved with biological limitations, machine intelligence can rely on high-bandwidth communication and silicon-based uniformity.
World Models and Accelerating Discoveries
To increase our progress, we need to explore two main avenues: using world models and using search to discover new phenomena. Humans have traditionally used technologies like microscopes, telescopes, particle accelerators, gene-sequencing computers, and wet labs to conduct new experiments. But how could superintelligent machines use these tools to accelerate research and make new scientific breakthroughs?
1. Simulated Environments
One approach is to use simulated environments where AI agents can conduct experiments using digital tools. However, physics-based simulations are often challenging to build, and they always have a "simulation to reality" (sim2real) gap. The reality is that we still do not fully understand the phenomena we wish to simulate—whether it be the intricacies of the brain, the mysteries of black holes, or the paradoxes of quantum physics. Creating a perfect simulator is an unattainable ideal.
2. Designing and Building New Tools
Another possibility is for AI agents to design new tools, which humans then build in the physical world. By bridging the gap between digital design and physical realization, we could leverage AI's innovative power to create more effective research tools.
3. Access to Physical Tools
A third possibility is to give AI access to the tools and technologies that humans already use. This would empower AI agents to acquire more direct agency in scientific research and pursue long-term projects. However, this approach is currently limited by the physical constraints of our world—our tools are bounded by spacetime and by the capabilities of the physical technologies that we have available on Earth.
The Current Challenge
We find ourselves in a paradoxical situation. AI agents cannot effectively use simulations to experiment with phenomena that are poorly understood, and they also do not have access to physical tools to bridge these knowledge gaps. This “dead end” highlights the importance of continued collaboration between human researchers and AI systems.
To make progress, we need better simulations, new physical tools, and more intelligent integration between AI-driven hypotheses and physical experiments. Only then can we advance from learning from our existing data to uncovering new truths about our universe.
Conclusion
“Learn and Search” encapsulates the next frontier in AI and scientific discovery. By leveraging the power of learning from vast amounts of data and utilizing intelligent search to explore new hypotheses, we have the potential to discover entirely new sciences and technologies. Though challenges remain—especially in the gap between simulations and reality—our progress thus far is proof that we are moving in the right direction.