December 2024
From Games to Reality: Learning World Models for Physical Intelligence
From AlphaGo to Generated Physical World Simulations
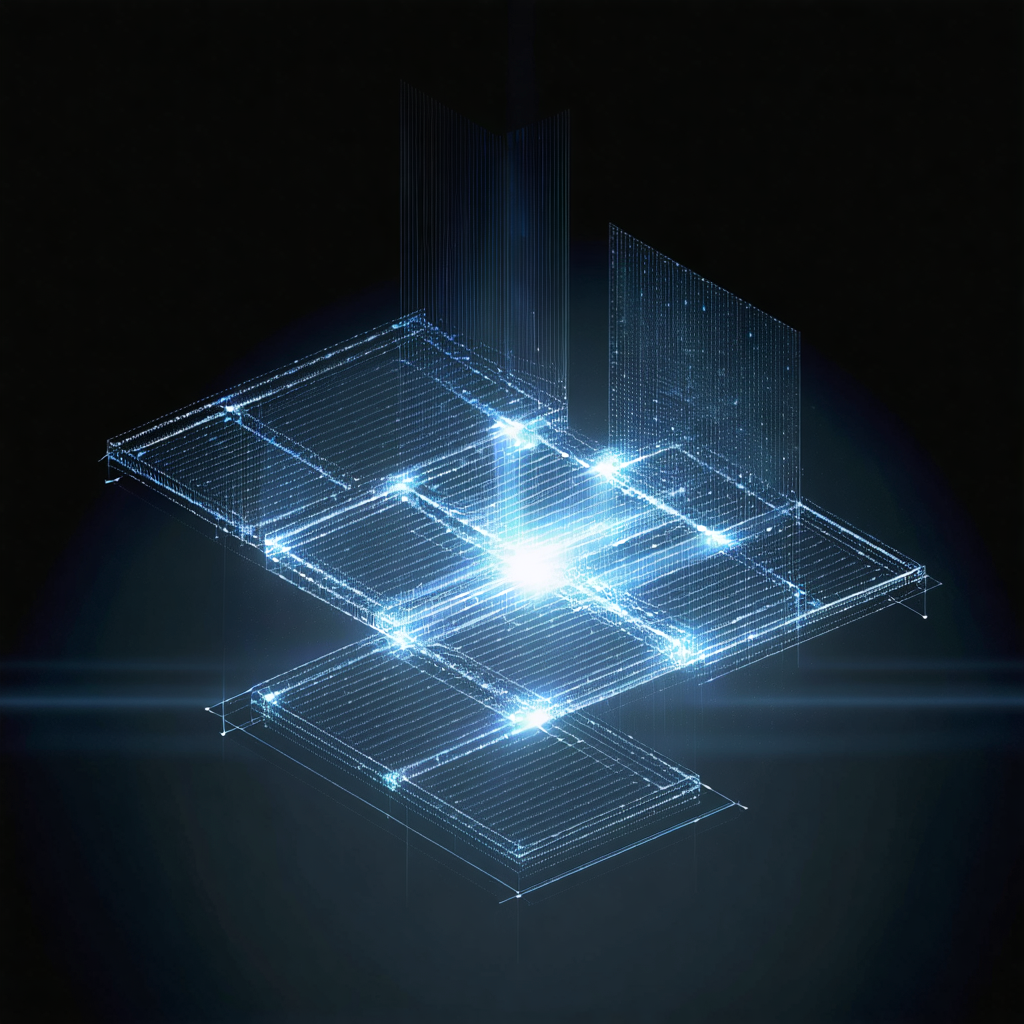
Listen to the Podcast Version
Introduction
At NeurIPS 2024, Sherry Yang presented a fascinating parallel between two transformative moments in AI. The first was AlphaGo's breakthrough in perfect-information games, where the ability to simulate and plan in a well-defined space led to superhuman performance. The second was GPT's mastery of language, where internet-scale learning enabled sophisticated understanding and generation of text. Now, we stand at the threshold of a third moment: the emergence of world models that can understand and reason about the physical world through video.
Learning from Perfect Information to Physical Reality
AlphaGo's success came from its ability to simulate future game states and plan optimal moves through tree search. However, unlike the perfect information environment of Go, the physical world is messy and unpredictable. Similarly, while language models have mastered the digital world of text, they struggle to capture the intricate physical dynamics of real-world interactions.
Yang's vision suggests a solution: just as language models learn from internet-scale text data to understand the digital world, video generation models can learn from vast visual data to understand the physical world. The key insight is that video, like text, can serve as a universal interface for capturing and reasoning about real-world dynamics.
From Text to Video: Parallel Learning Paradigms
The parallel between text and video world models runs deep:
- Scale of Data: Just as language models leverage Wikipedia and web text, video models can utilize YouTube and internet-scale visual data
- Unified Interface: Like text generation serving as a universal task interface for language, video generation can unify diverse physical world tasks
- Planning Capability: Similar to how language models can plan in text space, video models can plan physical actions through visual simulation
However, video world models offer unique advantages in capturing information that text cannot express: physical dynamics, spatial relationships, and detailed motion patterns.
Bridging Simulation and Reality
Unlike AlphaGo's perfect simulator or language models' digital domain, physical world models must contend with reality's complexities. Yang's approach addresses this through a three-part strategy:
- Learn broad world dynamics from internet-scale video data
- Enable planning through learned video simulation
- Ground predictions in reality through physical interaction
This combination allows systems to leverage the breadth of internet knowledge while maintaining accuracy through real-world feedback.
Conclusion
Yang's vision points to an exciting convergence of AlphaGo's planning capabilities and language models' learning from internet-scale data. While challenges remain - particularly around simulator imperfections and the need for physical grounding - the path forward is clear. By learning world models from video data and combining them with physical interaction, we're approaching a new paradigm in artificial intelligence: one that can understand, reason about, and interact with the physical world as fluently as AlphaGo plays Go or GPT models process text.